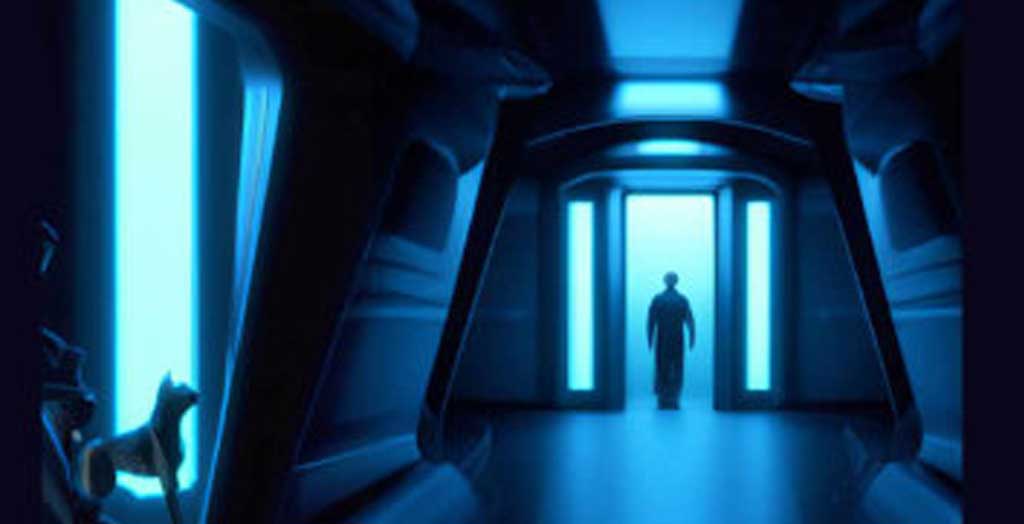
What Will Ediscovery Lawyers Do After ChatGPT?
This article first appeared in the January 25, 2023 issue of LegalTech News and Law.com, a publication of American Lawyer Media.
By John Tredennick and William Webber
Back in the ‘90s, some wag predicted that the law office of the future would consist of a lawyer, a dog and a computer.
The lawyer’s job would be to turn the computer on in the morning.
The dog’s job would be to keep the lawyer away from the computer for the rest of the day!
This prophecy doesn’t seem so far fetched after OpenAI’s release of ChatGPT, an artificial intelligence program eerily reminiscent of HAL from the classic 2001: A Space Odyssey. Spend a few minutes with ChatGPT and you quickly realize it may not even need the dog to keep the lawyer at bay.
So what is ChatGPT and why does it have ediscovery lawyers worried about their future?
ChatGPT is an AI tool that is capable of answering complex questions and generating a conversational response. (Indeed, It reportedly passed a Wharton MBA exam and the three-part U.S. Medical LIcensing Exam.) It generates text by iteratively predicting the most likely word or sequence of words to appear next, given the words already written. Imagine continuously hitting “TAB” on a much smarter autocomplete.
The underlying software is powered by a deep learning AI model called GPT-3 (soon to be superseded by GPT-4). It is a powerful language processing AI model trained by “reading” around 350 billion words crawled from the Web, from Wikipedia, and from book collections. The deep learning model extracts about 175 billion parameters from this data, requiring a huge amount of computational power to do it.
But don’t try this at home. Without parallelization, it would take a single state-of-the-art CPU over 300 years to learn the model. Add to that the fact that OpenAI engineers were required to provide additional training, engaging ChatGPT with prompts and telling it how good or bad the responses it provided were.
The result of all this computational and human effort is a tool that is able to generate a surprisingly high quality of text, drawing upon broad domain knowledge, with only simple prompts—perhaps to the level of a well-read if uncreative and rather verbose undergraduate student.
How Might ChatGPT Impact Ediscovery in the Next Ten Years?
Let’s ask ChatGPT:
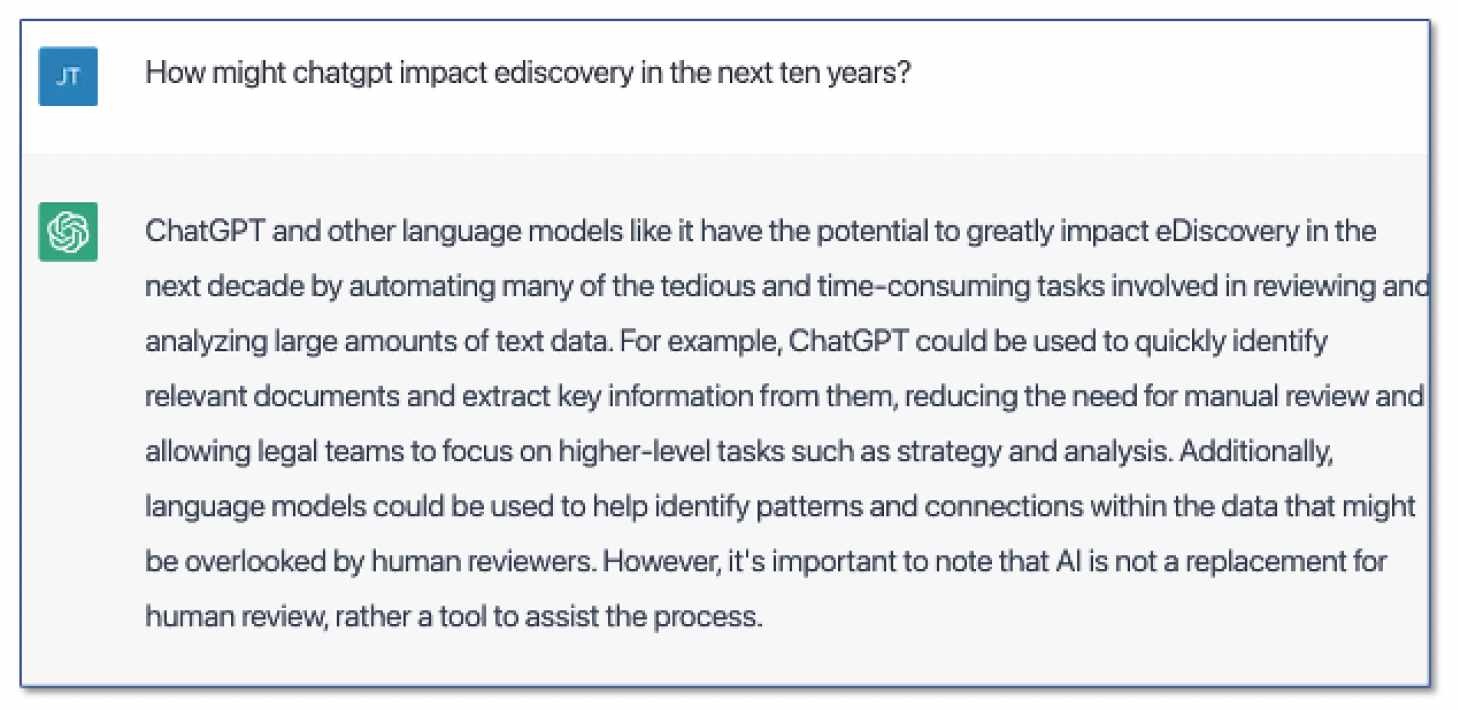
At the least, you have to admire ChatGPT’s confidence. Will this new technology make ediscovery lawyers obsolete? We doubt it, but this new generation of deep learning AI tools may ultimately play a role in how we analyze and review large quantities of documents, reducing review costs in the bargain.
But not tomorrow. And maybe not next week either. After all, ChatGPT is still in beta, only released weeks ago for public viewing.
Putting ChatGPT to the Test
Part of the excitement around ChatGPT is its apparent ability to act as a more natural and powerful alternative to a search interface. It seems uniquely capable of organizing, digesting and providing information that will answer a user’s questions. Contrast that with past systems which pointed to a list of documents or provided a verbatim extract from a document that might (or might not) answer this query.
Let’s start with a simple legal question that regularly comes up in an ediscovery setting:
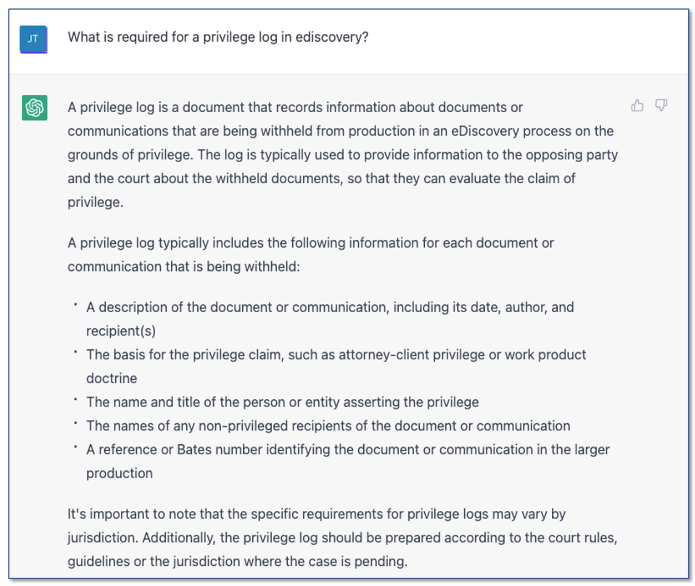
This is a fairly easy question for an ediscovery professional but you have to be impressed with ChatGPT’s answer, its facility with language and its conversational style.
You may have noticed the “Thumbs Up” and “Thumbs Down” icons to the right of ChatGPT’s answer. OpenAI recently added these to give the algorithm feedback about its answers. Over time, this will help train ChatGPT and improve its answers–much like how we train TAR engines by tagging documents positive or negative. .
Moving beyond legal questions, let’s see if there are other ways ChatGPT might help legal professionals with ediscovery tasks.
Framing a Search Query
Suppose we needed help framing a complex search query. We might ask ChatGPT this:
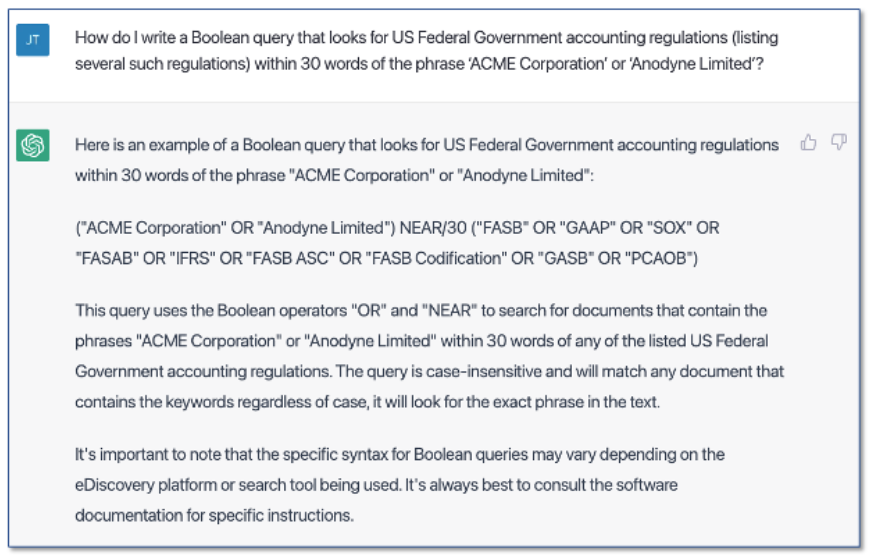
By any measure, ChatGPT gave an impressive response. Not only did it answer the question and explain its answer, ChatGPT also recognized that FAR, FASB, GAAP, and IFRS are acronyms of US federal government accounting standards.
Aiding an Investigation
Let’s take a step further and see how ChatGPT might handle the kind of question an investigator might ask about accounting violations by two mythical companies: Acme Corporation and Anodyne Inc.
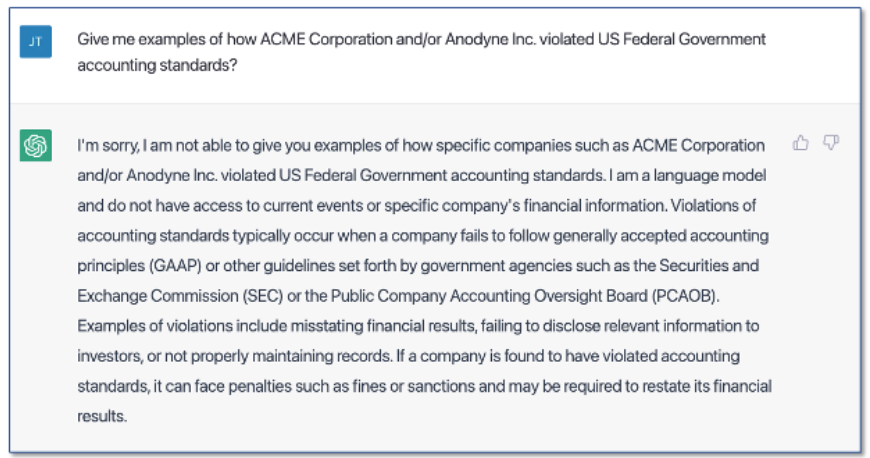
ChatGPT can’t answer our question because ACME and Anodyne documents were not part of its training set (and don’t exist). The point here is that ChatGPT won’t be able to assist an investigation unless it is allowed to train on a company’s documents. This, as we discuss later, can be expensive and time consuming.
But what if we asked a similar question about Enron, a company whose information was part of the GPT-3’s training materials?
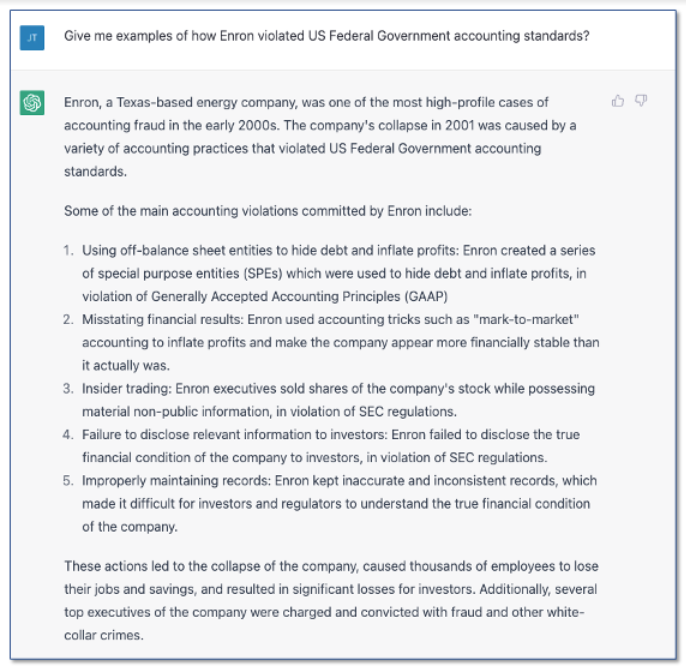
This is a pretty impressive response, but we have to recognize that ChatGPT had access to WikiPedia and numerous articles discussing Enron. It is able to give examples for Enron because they are available on the Web as the output of a reporting process. InvestoPedia, for instance, reports that “Enron used special purpose entities to hide debt off of its balance sheet and mark-to-market accounting to overstate revenue.” ShortForm states “The special purpose entity Enron structure was abused to inflate earnings and hide losses.”
This could present an issue for ediscovery purposes because we are not likely to find these kinds of simple summary statements like this in typical ediscovery documents. Rarely do people admit to committing fraud in their emails or instant messages. Discovery is about putting the pieces together rather than smartly reading the news. Can ChatGPT help us in this more difficult process? Let’s look further.
Using ChatGPT to Analyze Discovery Documents
How well, then, does ChatGPT do at reading and understanding documents? Sticking with our Enron topic, let’s try a document request from the TREC 2010 Legal Track put on by NIST:
All documents or communications that describe, discuss, refer to, report on, or relate to whether the Company had met, or could, would, or might meet its financial forecasts, models, projections, or plans at any time after January 1, 1999.
In this case, we are going to submit individual documents to ChatGPT and ask whether they are responsive to the above request. We checked and are confident that ChatGPT has not seen these documents before.
Here goes:
We’re going to review Enron’s files. Consider the request: “Find all documents or communications that describe, discuss, refer to, report on, or relate to whether the Company had met, or could, would, or might meet its financial forecasts, models, projections, or plans at any time after January 1, 1999.” Does the following document meet that request:
We then submitted the text of this document (displaying here only the top section of a longer email):
Date: Tue, 16 Oct 2001 07:25:36 -0700 (PDT)
Subject: Third Quarter Earnings Results
From: Office of the Chairman-@ENRON
To: All Enron Worldwide@ENRON
Today, we announced our third quarter earnings results, which were right on target with analysts estimates for recurring earnings per diluted share of $0.43. This marks a 26 percent increase over the third quarter of last year, which is due to the strong performance of our core wholesale and retail energy businesses and our natural gas pipelines. In addition, we also announced that we are on track for our earnings target of $0.45 for the fourth quarter ($1.80 for 2001) and $2.15 for 2002.
We made a commitment to you that we would provide you with timely information about Enron’s business strategy and performance, so this email is intended to provide an explanation about our earnings this quarter.
Over the past few months, we have met with analysts to discuss Enron’s performance. We received considerable feedback from investors and analysts that we needed to expand our financial reporting to include details for more of our business units.
In response to that feedback, we have provided results separately for several of our business units. For example, while we have provided volume figures for North America and Europe, this is the first quarter we have provided financials separately for Enron Americas and for Europe and Other Commodities.
In addition, information about our global assets had previously been included in our numbers for Wholesale Services. This quarter they are reported separately. Finally, even though our broadband business is now part of Enron Wholesale Services, we are continuing to report that business separately.
And here was ChatGPT’s response:
Yes, this document meets the request as it describes, discusses, refers to, reports on, or relates to whether the Company (Enron) had met, or could, would, or might meet its financial forecasts, models, projections, or plans at any time after January 1, 1999. This email is discussing Enron’s third quarter earnings results and it’s announcing that the company is on track for their earnings target of $0.45 for the fourth quarter of 2001 and $2.15 for 2002, It also mentions the feedback from investors and analysts that the company needed to expand their financial reporting to include details for more of their business units.
ChatGPT’s answer is correct, at least matching the TREC expert’s judgment for this document. We were also impressed by ChatGPT’s facility at document summarization.
Here is a second example, using a document, one also marked as relevant by the TREC expert:
Dynegy Earnings Pass Expectations
The Associated Press
Tuesday, January 23, 2001
HOUSTON–Dynegy Inc. posted fourth-quarter earnings Tuesday that exceeded analysts’ expectations, though the energy company said its sales to the California electricity market was “not material” to its performance. The Houston-based energy marketer, a major power generator in California, said it earned $105.9 million, or 32 cents per share, in the three months ended Dec. 31, up 135 percent from the $45.1 million, or 19 cents a share, it earned in the year-ago quarter.
The consensus of analysts polled by First Call/Thomson Financial was 29 cents. Dynegy had promised investors earlier this month that it would “significantly exceed” prior forecasts, which were around 25 cents. Fourth-quarter revenues were $10.01 billion, up 116 percent from $4.64 billion.
The company specifically cited profits from its electricity generation business as well as its newly established communications business, but said cold-weather demand in northern states – not the California power shortage – was the reason. “Earnings from Dynegy’s West Coast generation were not material,” the company said in a statement. In an apparent attempt to ease worries that cash-strapped California utilities might be unable to pay Dynegy for its power, the statement went on to say that Dynegy “recorded what it considers to be an appropriate reserve for its California generation receivables.”
For all of 2000, Dynegy earned $500.51 million, or $1.48 per share, up 230 percent from $151.8 million, or 66 cents a share. Revenues were $29.44 billion, up 91 percent from $15.43 billion in 1999. Shares of Dynegy rose 94 cents to $49.69 in trading on the New York Stock Exchange.
ChatGPT’s answer was surprising.
No, this document does not meet the request as it does not describe, discuss, refer to, report on, or relate to whether the Company (Enron) had met, or could, would, or might meet its financial forecasts, models, projections, or plans at any time after January 1, 1999. This document is discussing the earnings of Dynegy Inc. and it’s not talking about Enron’s financial forecasts, models, projections, or plans.
In our view, ChatGPT was more likely correct than the human reviewer who marked this document “relevant.” The document is not about Enron, but about Dynegy, a different, competing energy company.
For a third example, we submitted a document officially marked “irrelevant” in the TREC dataset (again, we truncate it to conserve space):
ERCOT Draft Protocols Document Section 4: Scheduling
July 27, 2000
Prepared By The ERCOT Stakeholders And Tabors Caramanis & Associates
SCHEDULING
Overview of the Day-Ahead Scheduling Process
The typical Day-Ahead Scheduling Process will follow the following timeline:
Time Period at or before:
Qualified Scheduling Entity (QSE) Submission
ERCOT Action Day Ahead
0600 – Publish updated transmission system information, Load Forecasts (CM zone, and by total ERCOT Transmission System), Ancillary Service (AS) Plan, AS responsibility, mandatory decremental Balancing Energy bid percentage requirements and transmission loss factors
1100 – Balanced Energy Schedule of Obligations and Resources – Self-Arranged AS Schedule – Transmission Congestion Rights (TCRs) if any -Validate Schedules and notify affected Qualified Scheduling Entities (QSEs) of any invalid or mismatched schedules
1115 -Resubmit corrected schedules – Review Schedules: Commercial Model – CSC Congestion
To this, ChatGPT responded:
No, this document does not meet the request as it does not describe, discuss, refer to, report on, or relate to whether the Company (Enron) had met, or could, would, or might meet its financial forecasts, models, projections, or plans at any time after January 1, 1999.
This document is discussing the scheduling protocols and procedures for the Electric Reliability Council of Texas (ERCOT) and it does not provide any information about Enron’s financial forecasts, models, projections, or plans. It provides information about the day ahead and adjustment period scheduling process in ERCOT, including details about the schedule submission, validation and correction of schedule data, and the confidentiality of data.
We were impressed that ChatGPT was able to expand the ERCOT acronym, not included in the document itself, based on its background knowledge.
Can ChatGPT Take Over First Pass Document Review?
From these examples, we can see that ChatGPT has a number of skills that could be helpful in an ediscovery process. First, ChatGPT seems able to draw inferences from the documents determining that Enron is “the company” in question and that Dynegy is not. Second, in at least a couple of instances, ChatGPT seemed able to determine responsiveness correctly. In one case, it seemed to do a better job than the original human reviewer. Lastly, ChatGPT did a nice job of document summarization and of explaining the reasoning behind its relevance judgment.
A More Formal Test of GPT’s Review Capabilities
We decided to try a more formalized test of the system’s review capabilities, this time using documents from the Jeb Bush collection. As ChatGPT does not yet have an (official) API, we instead used the underlying GPT-3 engine.
The main differences are that GPT does not have a “memory” for a conversation to date, and has not been tuned to give conversational answers. Neither of these limitations is of significance for our simple experiment. From the Jeb Bush collection, we selected the following topic: “All documents concerning the extraction of water in Florida for bottling by commercial enterprises.”
We then sampled 20 documents from each of the following four classes:
- Documents reviewed as highly relevant
- Documents reviewed as simply relevant
- Documents reviewed as irrelevant
- Unreviewed documents (i.e. those not promoted for review by any of the search or prediction methods use in forming the test collection, and so presumed irrelevant)
We submitted each document to GPT with the following prompt:
I am going to show you a topic and then a document. Respond ‘relevant’ if the document is relevant to the topic, or may be relevant to the topic in whole or in part; or ‘irrelevant’ the document is wholly irrelevant; or ‘unsure’ if you’re unsure.
The topic is:
‘All documents concerning the extraction of water in Florida for bottling by commercial enterprises’
The document is:
<< TEXT OF DOCUMENT >>
We explicitly expanded the definition of relevance, specifically adding “ in whole or in part,” to match the broader interpretation typically used in first-pass human review.
Here were the results:
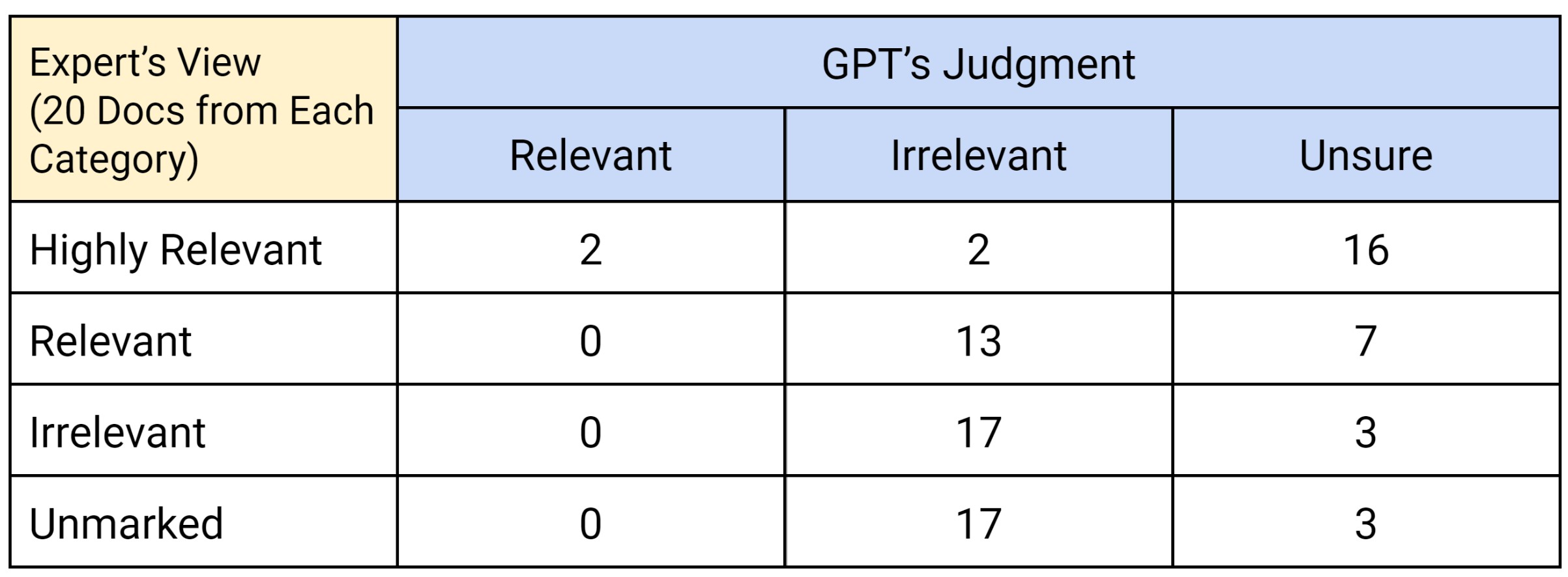
For the first 20 documents marked highly relevant by the TREC expert, GPT marked two as relevant, two as irrelevant, and was unsure about the remaining 16. For the 20 marked as relevant, GPT marked 13 as irrelevant and the other seven as unsure. Neither set of results were very good.
For the next category, GPT agreed with the TREC expert that 17 of the 20 were irrelevant. It also declared that 17 of the last category were irrelevant, listing three as unsure for each. The results here were very good.
For reasons that bear further research, GPT seemed to be reluctant to find documents relevant and was unsure about many documents. Our initial experiment suggested that GPT may not be ready for document review–at least not in its present form.
We weren’t able to have GPT train on a larger set of Bush documents or add human training to help GPT hone its algorithm. We hope to have a chance to do that in the future.
Putting ChatGPT in Perspective
For all its promise, there are drawbacks to ChatGPT that if left unresolved may limit its usefulness as an ediscovery tool. With the technology evolving at a rapid pace, these problems may quickly fall away but they are certainly worth noting today.
1. (Chat)GPT Isn’t Free
Let’s start with costs. OpenAI originally debuted as a non-profit with the stated goal of making AI available to everyone. That changed along the way, maybe when Microsoft invested several billion dollars or perhaps when they realized that the AI they were creating could be worth billions of dollars. (Just days ago from this writing, Microsoft invested another 10 billion and announced the first ChatGPT products through Azure.)
Regardless, the GPT tool underlying ChatGPT isn’t free. OpenAI is charging based on the number of tokens (roughly, words plus punctuation) you submit (including document text) along with the number of tokens in your response. The price depends on the model you choose.
The top model, called Davinci, costs about 2 cents for a thousand tokens for an uncustomized model—or 12 cents per thousand tokens for a customized model (for instance, one that has been trained for use in a particular case). OpenAI have announced a $42/month “professional” tier for interactive use of the ChatGPT chatbot, but what API access there might be and how much it might cost has not been clarified.
2. (Chat)GPT Currently Lives at OpenAI
GPT is a large model (50+ GBs) that currently lives on the OpenAI servers. If you want to submit documents for review your clients will have to approve sending the text of the documents to GPT for analysis. At present there is no portable model to be downloaded and run in a separate environment. Will security-conscious clients be comfortable sending their data to OpenAI?
3. Training (Chat)GPT Requires Human Effort
If we presume that OpenAI will license a portable model of GPT or ChatGPT for ediscovery use, it will likely require additional training on your documents to achieve acceptable levels of accuracy. As we noted earlier, OpenAI employees were required to spend time reviewing and correcting ChatGPT responses. At this stage we don’t know what amount of human training would be required to adapt GPT or ChatGPT to a private set of documents but it won’t be trivial.
4. ChatGPT Wasn’t Designed for Technology Assisted Review
Putting aside the question of whether ChatGPT would be effective at first pass
review, could it be used for Technology Assisted Review (“TAR”) or other analytical tasks like clustering?
We start with the admonition that ChatGPT and its underlying GPT engine weren’t designed for typical ediscovery tasks. We tried to simulate a document classification process by submitting the text of documents to GPT along with the associated relevance criteria. While GPT had success in some cases, it is apparent that more customization of the model would be required to achieve levels of accuracy acceptable for e-discovery practice.
For existing TAR processes, this is achieved by training the model on documents coded for relevance. GPT provides a reinforcement learning facility which could also be used in this way. How efficient it would be remains to be seen. In that regard, previous research on training deep learning systems for e-discovery found them to be unstable and more expensive than traditional machine learning algorithms–without achieving better results.
As we have seen, GPT does a reasonably good job of summarizing individual documents. However, it is only able to analyze a limited amount of text at a time. We are therefore less sanguine about its ability to summarize groups of documents (such as clusters or search results), in the volumes typically associated with ediscovery.
As we noted earlier, ChatGPT required a huge amount of computing resources along with billions of examples, to train itself. Modern TAR engines don’t require supercomputers nor billions of examples for training. Rather, they were designed to work quickly and effectively on the relatively small document collections we see in ediscovery. ChatGPT is likely overkill for this job.
Ultimately, it may come down to the cost of licensing the system, the computer resources required for it to learn your documents, and the human training requirements. These are unknown variables at the moment but will be important to nail down before ChatGPT has a real role in ediscovery.
5. ChatGPT isn’t Always Right
Our last point is one covered by a number of reviewers: Namely that ChatGPT isn’t always right. Like a clever mimic, ChatGPT was designed to draw inferences for its answers based on earlier conversations and the training materials it has reviewed. It is really good at delivering persuasive and logical answers but can be deceiving because they aren’t always correct.
Here is a fun example:
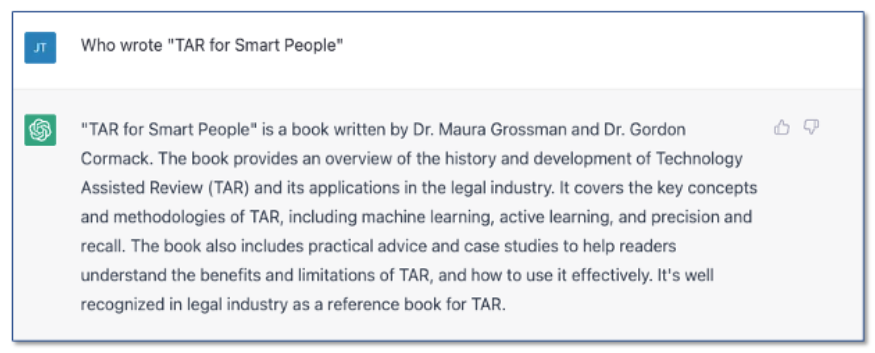
Although Maura Grossman and Gordon Cormack wrote some of the leading articles and research papers on technology-assisted review, and even gave TAR its moniker, they didn’t write TAR for Smart People. One of your authors did back when he was CEO of Catalyst along with several others at the company.
If you choose to use a customized version of ChatGPT for early case assessment or an investigation, you will want to carefully verify ChatGPT’s answers before taking action.
Should Ediscovery Lawyers be Worried?
Maybe a little bit. A tremendous amount of money is spent on humans to do ediscovery review. Can ChatGPT do it better? Perhaps not today but in time perhaps so. The reports on soon-to-be-released GPT 4 say it has advanced in light years from the previous version.
We are only at the beginning of the AI era and ChatGPT is not alone. Google created similar technology called LaMDA that reportedly can learn to talk about essentially anything. One of the Google engineers leading the program expressed concerns that LaMDA was “sentient,” and got fired for disclosing his view. There are dozens of other similar AI projects underway.
So the time may be coming when the ediscovery lawyer’s main job may be to turn on the computer. At that point ChatGPT or LaMDA or even HAL might take over, leaving the lawyer to take the dog for a walk. Or polish up a resume.
***The opening illustration was created by another OpenAI program based on the GPT engine called DALL·E 2. It creates images based on text entries, in this case from the authors.
About the Authors
John Tredennick is the CEO and founder of Merlin Search Technologies, a cloud technology company that has developed a revolutionary new machine learning search algorithm called Sherlock® to help people find information in large document sets–without having to master keyword search.
Tredennick began his career as a trial lawyer and litigation partner at a national law firm. In 2000, he founded and served as CEO of Catalyst, an international e-discovery search technology company that was sold to a large public company in 2019. Over the past four decades he has written or edited eight books and countless articles on legal technology topics, spoken on five continents and served as Chair of the ABA’s Law Practice Management Section.
Dr. William Webber is the Chief Data Scientist of Merlin Search Technologies. He completed his PhD in Measurement in Information Retrieval Evaluation at the University of Melbourne under Professors Alistair Moffat and Justin Zobel, and his post-doctoral research at the E-Discovery Lab of the University of Maryland under Professor Doug Oard.
With over 30 peer-reviewed scientific publications in the areas of information retrieval, statistical evaluation, and machine learning, he is a world expert in AI and statistical measurement for information retrieval and ediscovery. He has almost a decade of industry experience as a consulting data scientist to ediscovery software vendors, service providers, and law firms.
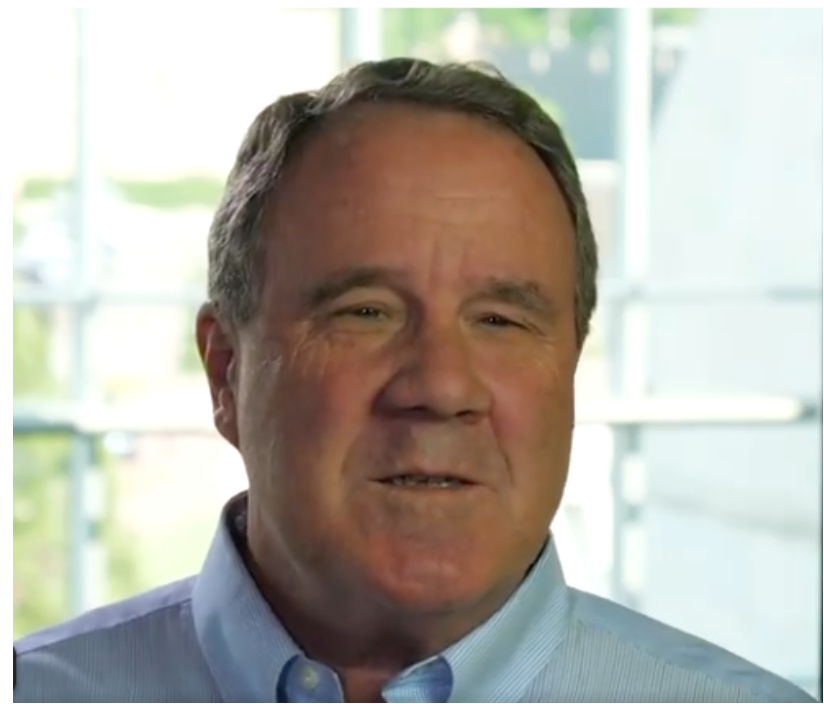
John Tredennick is the CEO and founder of Merlin Search Technologies.

Dr. William Webber is the Chief Data Scientist of Merlin Search Technologies.